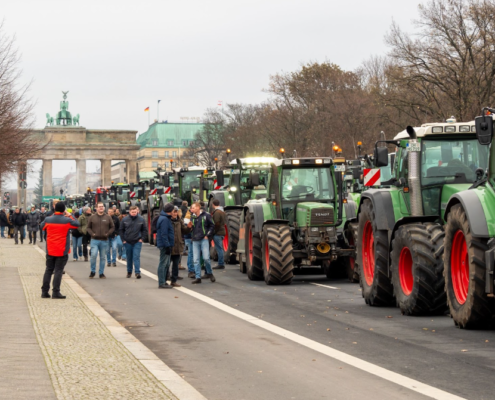
July 7, 2023
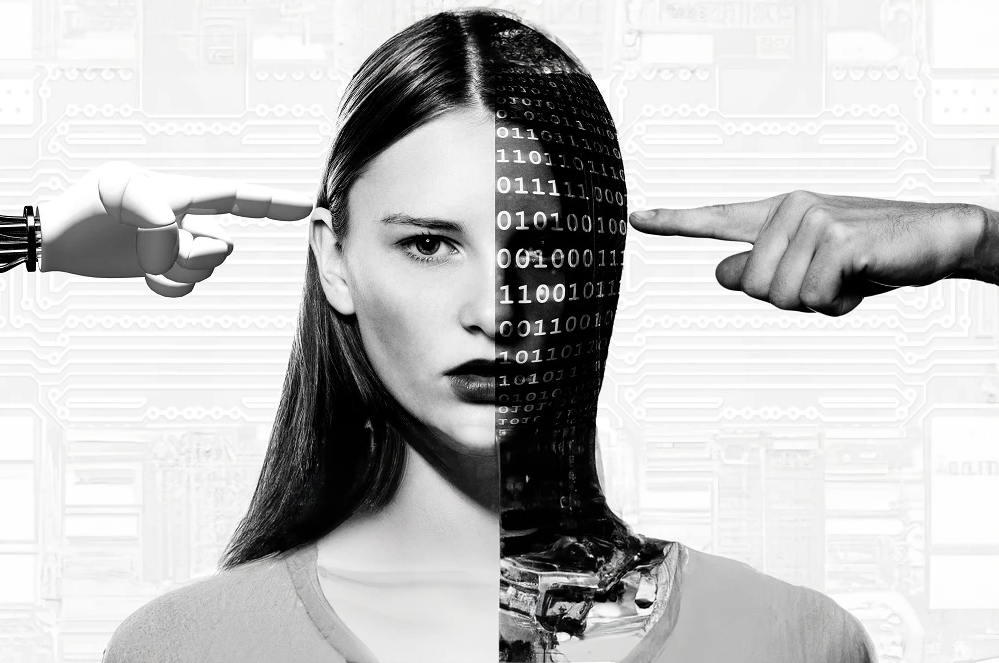
Artificial intelligence (AI) has gradually become more prevalent in our daily lives. We ask Siri about the weather or have Chat GPT type our work emails. But what does it take to make all these AI-driven devices work?
The hidden work behind AI
First things first: what we call ‘artificial intelligence’ today is neither artificial nor intelligent. Indeed, early AI systems were strongly driven by rules and programmes, but today’s systems (including the beloved ChatGPT) do not rely on abstract rules. Instead, it claims the work of real people – artists, musicians, programmers and writers – in the name of saving civilization. At best, this can be called ‘non-artificial intelligence’ [1].
“What we are witnessing is the wealthiest companies in history (Microsoft, Apple, Google, Meta, Amazon …) unilaterally seizing the sum total of human knowledge that exists in digital, scrapable form and walling it off inside proprietary products, many of which will take direct aim at the humans whose lifetime of labor trained the machines without giving permission or consent.”
Naomi Klein, Professor of Climate Justice [2]
The (big) data of these and many other people are like fuel for AI systems, with the Internet of Things (IoT) being a gas station providing exponentially increasing amounts of data. At the same time, the dimensionality of the data (the quality of having many different features) has also been expanded. These large amounts of high-dimensional data make the data more comprehensive and sufficient to support AI development [3].
AI programmers discovered that larger datasets could generate more interesting and smarter outcomes, thus moving to massive, automatically collected datasets like the Common Crawl [5]. The underlying sources, however, are full of racism, sexism and homophobia, along with other ideologies and social orientations that are unacceptable today. These databases require extensive filtering to match the sensibilities and moral agendas of our time and as a necessary corrective to many existing prejudices [4].
Ghost workers
Training and filtering of datasets cannot be done automatically. This work is outsourced to “ghost workers” hired by BPOs (Business Process Outsourcing companies, red). They transcribe conversations, review images, and label, categorize and clean up data. Despite their crucial role, they usually earn less than the legal minimums wage, with no health benefits, and risk being fired at any time [5].
“[Big Tech] companies are very reluctant to disclose the mere presence of human workers. They don’t disclose the presence of data workers, they don’t talk about how much these workers are getting paid, where they are, and under which conditions they work. […] Our fancy new chatbot is trained on the labour of workers in Syria, who not only live in a war-rhythm place, but also they are paid by task. They never know what they will make at the end of the month. There is no way for these workers to tell us we did them wrong. Who wants to disclose that? Nobody.”
Milagros Miceli, sociologist and computer scientist [6]
Ghost workers exposed to psychologically disturbing content sometimes work up to 10 hours daily. Psychological support seems to fall short, as the client’s interests tend to prevail:
“You are able to take well-being time. They tell us to take as much as we need, but on the other hand, we have key performance indicators. I have to fulfil these targets and stay in production. They do not argue about mental health. They don’t care”.
Anonymous ghost worker in Germany [6]
Meanwhile, The Washington Post revealed that the content of numerous pornographic, white supremacy, anti-immigration websites were fed to AI systems. Even the content of the anonymous message board 4chan.org was used, which is known for organizing targeted harassment campaigns against individuals. Anti-Muslim bias also emerged as a problem in some language models.
“Systematic systems tend towards a kind of statistical average. We move towards the greatest common denominator, the statistical mean. Then we lose all the nuances. Everyone who is different adds to the nuance. Systematic systems tend towards a kind of statistical averaging.
Vladan Joler, professor of new media [6].
Hence, stereotypes are genuinely ingrained in automatically collected datasets. The harms, biases and injustices resulting from algorithmic systems vary and depend, among other things, on the training and validation data used, the underlying design assumptions and the specific context in which the system is used. However, one thing remains constant: individuals and communities at the margins of society are disproportionately affected [7].
“AI will solve the climate crisis”
We already saw that AI has many social implications. How about the environment? Proponents argue that AI could combat climate change, referring to the potential for mitigation (e.g. measurement, reductions and removal) as well as adaptation and resilience (hazard forecasting and vulnerability and exposure management) [8]. A study from accounting firm PwC commissioned by Microsoft claimed that AI could facilitate a 4% reduction of total GHG emissions in 2030, whereas the Boston Consultancy Group estimated it at 5-10%.
With the number of calculations to improve AI systems constantly increasing, the amount of computation has been doubling every six months in recent years, compared to every 18 months at the beginning [6]. The production of chips and semiconductors required to keep up with the increasing computing demand is highly energy-intensive, expensive and has a carbon impact at every step. The rise of computing is also reflected in significant growth in data centers. The power consumption and CO₂ emissions from such centers doubled between 2017 and 2020 [9].
According to Vladan Joler, one model like ChatGPT-4 requires about 25,000 chips to work, with the next generation requiring about 100 times as many. The training of ChatGPT-3 alone caused about 550 metric tonnes of CO₂ – approximately 250 return flights between Amsterdam and New York [10]. Open AI, the company behind ChatGPT, refused to disclose how long and where its new GPT-4 has been trained or disclose anything about the data used, making it impossible to estimate emissions [11].
The amount of water consumed in creating and using AI models is even more unclear. Data centres use water in evaporative cooling systems to avoid overheating. The amount of freshwater needed to train GPT3 in Microsoft’s US data centres is estimated at 700,000 litres [11].
Naomi Klein concluded that AI is far more likely to be marketed in ways that actively exacerbate the climate crisis. The giant servers that enable instant essays and artwork from chatbots are a vast and growing source of carbon emissions. Moreover, she sees companies like Coca-Cola making considerable investments to use generative AI to sell more products. It becomes all too clear to her that this new technology will be used in the same way as the previous generation of digital tools: that what starts with lofty promises of spreading freedom and democracy ends in micro-targeted advertising so that we buy more useless, carbon-spewing stuff [2].
To live in a world of AI is to live in a world of statistical mediocrity. Do we want to live in that world, and who and what determines that? That the processes behind AI do not just fall from “the cloud” is given. It always comes with a price tag of blood, sweat and metals [6].
Related articles:
References:
[1] Morozov, E. (2023, 6 April). Artificial intelligence is not artificial. And certainly not intelligent (Translated by Author). Retrieved from https://decorrespondent.nl/14393/kunstmatige-intelligentie-is-niet-kunstmatig-en-al-helemaal-niet-intelligent/82ce3a36-0987-0f2a-2b01-91f2bdb92127
[2] Klein, N. (2023, 8 May). AI machines aren’t ‘hallucinating’. But their makers are. Retrieved from https://www.theguardian.com/commentisfree/2023/may/08/ai-machines-hallucinating-naomi-klein
[3] Zhang, C. & Lu, Y. (2021). Study on artificial intelligence: The state of the art and future prospects. Journal of Industrial Information Integration, 23, 100224. https://doi.org/10.1016/j.jii.2021.100224
[4] Olga, A., Saini, A., Zapata, G., Searsmith, D., Cope, B., Kalantzis, M., Castro, V., Kourkoulou, T., Jones, J, Da Silva, R. A., Whiting, J., Polyxeni, N. & Kastani, N. P. (2023). Generative AI: Implications and Applications for Education. https://doi.org/10.48550/arXiv.2305.07605
[5] Gray, M. L., & Suri, S. (2019). Ghost work: How to stop Silicon Valley from building a new global underclass. Eamon Dolan Books.
[6] Meerman, M. (editor-in-chief). (2023, 8 June). The price of AI (Translated by Author) [Documentary]. In Tegenlicht. VPRO. Retrieved from https://www.vpro.nl/programmas/tegenlicht/kijk/afleveringen/2023-2024/de-prijs-van-ai.html
[7] Birhane, A. (2021). Algorithmic injustice: a relational ethics approach. Patterns, 2(2). https://doi.org/10.1016/j.patter.2021.100205
[8] Maher, H., Meinecke, H., Gromier, D., Garcia-Novelli, M. & Fortmann, R. (2022, 7 July). AI Is Essential for Solving the Climate Crisis. Retrieved from https://www.bcg.com/publications/2022/how-ai-can-help-climate-change
[9] Jariwala, D & Lee, B. C. (2023, 8 March). The hidden costs of AI: Impending energy and resource strain. Retreived from https://penntoday.upenn.edu/news/hidden-costs-ai-impending-energy-and-resource-strain
[10] Luccioni, A. S., Viguier, S., & Ligozat, A. L. (2022). Estimating the carbon footprint of bloom, a 176b parameter language model. https://doi.org/10.48550/arXiv.2211.02001
[11] Singh, M. (2023, 8 June). As the AI industry booms, what toll will it take on the environment? The Guardian. Retrieved from https://www.theguardian.com/technology/2023/jun/08/artificial-intelligence-industry-boom-environment-toll
Cover and preview photo: The human side behind AI. Adjusted from free source photo by geralt on Pixabay.